Efficient Symbolic Approaches for Quantitative Reactive Synthesis with Finite Tasks
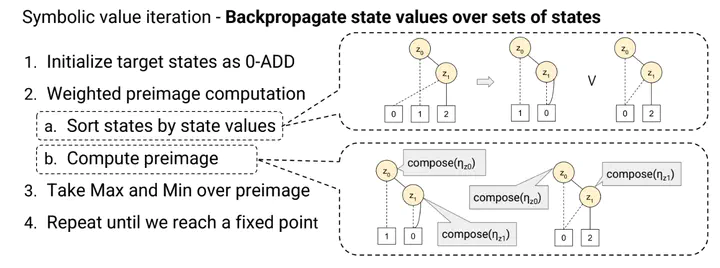
In this talk, we will introduce efficient symbolic algorithms for quantitative reactive synthesis using Algebraic Decision Diagrams (ADDs). We consider resource-constrained robotic manipulators that need to interact with a human to achieve a complex task expressed in linear temporal logic over finite trace (LTLf). Our framework generates reactive strategies that not only guarantee task completion but also seek cooperation with the human when possible.
We model the interaction as a two-player game and consider regret-minimizing strategies to encourage cooperation. We use symbolic representation of the game to enable scalability. For synthesis, we first introduce value iteration algorithms for such games with min-max objectives. Then, we extend our method to the regret-minimizing objectives. Our benchmarks reveal that our symbolic framework not only significantly improves computation time (up to an order of magnitude) but also can scale up to much larger instances of manipulation problems with up to 2x the number of objects and locations than the state of the art.